Cognitive musicology : A self-learning neuromorphic chip exhibits avant-garde application
- Saunak Saha
- Aug 6, 2018
- 4 min read
IMEC Technology Forum 2017, Antwerp, Belgium.
A simple demonstration of a novel computing prototype brought together the worlds of artificial intelligence and musicology and threw all their existing paradigms into question. Belgian International R&D establishment, IMEC just released their Self-Learning Neuromorphic Chip that uses OxRAM based neurosynaptic core to perform completely unsupervised learning of tasks literally mimicking the contemporary understanding of neural processing mechanisms. To be taken with a grain of salt, the chip therefore could harness the capability to crunch patterns in bulky data and subsequently infer classifications with minuscule power requirement, a unique characteristic of the brain that has baffled and eluded Von Neumann computing architectures for generations. On top of that, what makes this chip even more revolutionary is the ability to self-learn with no human supervision, something reminiscent of the mammalian prefrontal cortex.
But if that wasn't enough for you cynics out there, Praveen Raghavan - Distinguished technical leader of Neuromorphic computing group at IMEC, is certainly on board with you. Notwithstanding the plethora of socioeconomically relevant applications that could be used to demonstrate the chip, he arbitrated to kick it up a notch. Closely resembling the subversive nature of his innovation, he selected an artistic way of demonstrating the cognitive capability of this chip.
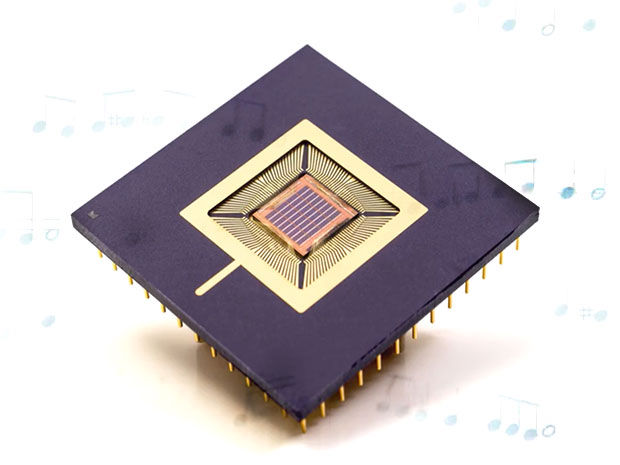
But before we get to that, let us put things into perspective. Neurons in our brain learn, infer, or in a nutshell, communicate using spikes. It is in the temporal distribution of these spikes, that meaningful patterns and messages are encoded. This is one of the primary reasons why neural computation is so thrifty in terms of power and resources. Whatever the source of sensory data, the messages are received embedded in monolithic voltage spikes. Music, therefore by instinctive argument, is perceived as auditory spikes to particular spatial distributions in our cortex. The neurons communicate via conducting ion-channels among them known as synapses to communicate these spikes and here is where the learning emerges. The neurotransmitter release, that is the principal contributor towards the relay of spikes through synapses is controlled by patterns. In other words, synapses are variable in their effectiveness of spike-transmission or 'Plastic'. Theoretical neuroscientists have classified this phenomenon into Short-Term or Long-Term (Spike Time Dependent) Plasticities according to the timescale on which they manifest themselves. Among our VLSI artillery, Resistive-RAM or RRAM is one of the leading weapons that can elicit synaptic behavior on-chip and naturally expose themselves to online unsupervised pattern recognition. Put an array of these crosspoint memory cells together with associated neural circuitry and you have a working Neuromorphic core primed in the crafts of machine learning. Unfortunately, I've managed to make it sound more facile than it ends up being.
But the challenge was not only aced but transcended by IMEC's bleeding-edge research. They trained a network of biologically plausible neurons, embedded as dynamically configurable neuron circuits in the chip, on auditory spikes from classical Flemish music. This included Belgian and French flute minuets having a consistency of musical style. This is because they wanted the chip to cognitively fish out patterns underlying the particular style of music. An example can be drawn from the ♭5th of the blues scale. A pattern can be quickly resolved if it closely follows the 4th in all of its example music pieces. But of course, to the neural processing unit, all this is promptly fathomed via spike patterns. As a result of being extensively trained on Flemish classical pieces, the chip marveled at discerning the note-by-note patterns, chromatic runs, time-signatures and whatnot associated specifically to the old Belgian minuet.
As a part of the inference phase of the demo, the learned synaptic states were fixed and the chip was allowed to emit spikes to an off-chip processor to transform the spike signatures back to auditory signal. Before I elaborate on my opinion about the performance of the chip, here is the actual demo released by IMEC:
In my neophyte grasp of classical music, I thought this was inspired, the first silicon virtuoso of its kind, worthy of being le chef d’orchestre. As an advocate of this field of engineering and as someone who conducts regular efforts to incrementally understand the principles of it, I welcome this innovation with all the enthusiasm I can possibly fathom. However, critically enough, the composition is typical of someone who is a beginner in this particular style of music. It almost feels like a pianist trying to recreate certain patterns of the staff rather than taking more unconventional pathways to a new tune. Even so, a few odd notes here and there keep our hopes of reviving a Vieuxtemps or a Franck within the confines of a circuit board.
Across the globe, scores of computer scientists are looking to create applications based singularly on one idea. The Human Brain. It is an exciting and exacting time to be a proponent of this field. In the face of such confounded affairs, IMEC bespoke a brilliant example with this chip. With all these applications, ever more demanding, we need the machinery to fuel the movement. It is time to bring down the proverbial wall between compute and memory and build equipment capable of crunching data rather than numbers, all with the expense of a lightbulb's worth of power.
Comentários